Building the Backbone of Enterprise AI: Tools, Infrastructure & Strategy
Practical insights on the infrastructure powering scalable AI at growth-stage companies
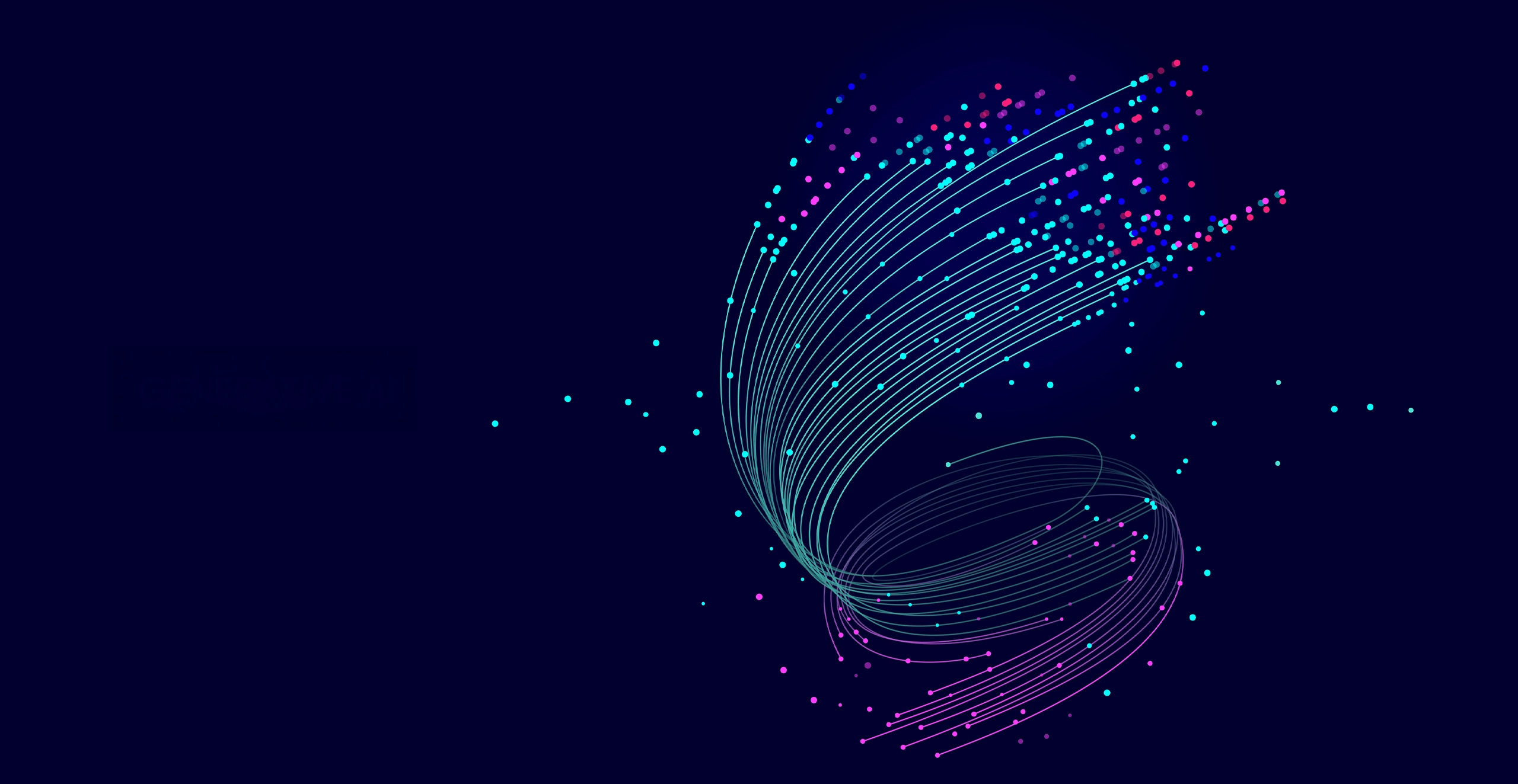
AI has moved from experimentation to execution. For growth-stage companies, the question is no longer whether to adopt AI — it’s how to do it in a way that creates lasting business value. We believe that begins not with the model itself, but with the AI infrastructure that supports it.
At Summit, we work closely with a broad range of portfolio companies to help integrate AI into their products and operations — from marketing tech to enterprise software to life sciences. What we see time and again is that success with AI doesn’t just depend on which model you use — it depends on the systems you build around it.
To unpack what we believe it takes to move AI from concept to impact, Sharon Lin, VP of Data Science at Summit, outlines five foundational categories helping to shape the future of enterprise AI infrastructure. Drawing on her deep experience in applied AI — and her work with technical and product leaders across the Summit portfolio — Sharon offers a practical guide to the technology stack behind enterprise-grade AI and the tools, layers and logistics that can turn models into meaningful business outcomes.
1. Semantic Layer and Vector Infrastructure for RAG & Embeddings
As foundation models become increasingly commoditized, the competitive edge is shifting to how companies structure, store and retrieve data. Technologies like Retrieval-Augmented Generation (RAG) and semantic search have emerged as mission-critical tools for grounding AI models in real-time, domain-specific context.
Embeddings encode meaning, and vector databases index and retrieve that meaning at scale. Together, they enable AI systems to deliver responses that are not just fluent but contextually relevant and actionable.
What this means for leaders: Companies that invest in a strong semantic layer can build systems that allow AI to tap into proprietary knowledge — helping to turn unique organizational data into a strategic asset and enabling more accurate and differentiated AI applications.
2. Hybrid and Multicloud AI Ops
The AI boom is both resource-intensive and geographically sensitive, forcing many companies to rethink their cloud infrastructure strategies. Hybrid and multicloud architectures are no longer just IT considerations — they are increasingly viewed as strategic tools for supporting scalable and compliant AI development.
Hybrid cloud setups allow organizations to combine the speed and security of on-prem systems with the flexibility of public cloud platforms. Meanwhile, multicloud approaches help optimize performance, access specialized AI services and avoid vendor lock-in.
What this means for leaders: Work closely with your technical teams to determine the right balance of cloud and on-prem infrastructure. The goal is to support scalable AI performance and cost-efficiency — without compromising compliance or flexibility for future growth.
3. Data Labeling, Synthetic Data & Reinforcement Learning (RLHF)
In AI, high-quality training data are both a company’s greatest asset and often its biggest bottleneck. While foundation models are increasingly available off the shelf, we believe true differentiated performance comes from domain-specific data.
To build this data layer, some organizations are turning to a combination of human-labeled datasets, synthetic data generation and reinforcement learning from human feedback (RLHF). Together, these methods enhance coverage, reduce bias and align AI outputs with real-world judgment.
What this means for leaders: Your proprietary data are a core part of your competitive advantage. By investing in high-quality, domain-specific data pipelines, companies can create a feedback loop designed to continuously improve AI performance and drive differentiation. Prioritizing the right data pipelines ensures your AI systems are purpose-built to reflect your business, your customers and your standards.
4. AI Compliance, Governance & Observability
As regulatory frameworks like the EU AI Act and emerging U.S. policies take shape, compliance is becoming a full-stack function. Tools that offer traceability — from data lineage to output decisions — are critical for building trust and minimizing risk.
Organizations need clear systems for tracking how data are collected, labeled, processed and used in AI models. This is particularly important in regulated industries like healthcare, finance and defense, where auditability is essential.
What this means for leaders: Treat AI governance as a business enabler, not a blocker. Building compliance into your stack from the start helps future proof your AI strategy, accelerate adoption and build long-term stakeholder confidence.
5. Agent Orchestration
As AI matures, companies are moving from single-purpose tools to coordinated systems capable of executing complex, multi-step workflows. This shift requires orchestration platforms that can manage interactions across multiple AI agents, maintain memory and context and integrate with external APIs or tools.
These platforms help automate everything from customer support to internal operations - improving consistency and scalability while reducing manual intervention.
What this means for leaders: Orchestration is likely to be key to turning isolated AI experiments into integrated business systems. Look for tools that support modular, reliable automation across your organization.
Growth Timeline
Don't delete this element! Use it to style the player! :)
.png)
In the rush to adopt AI, it’s easy to focus on headline-grabbing models and overlook the stack that makes them practical and scalable. But, as AI becomes part of core business operations, we believe long-term success will depend not just on the models companies choose, but by the enterprise AI infrastructure they build around them. For leaders, that means thinking not just about what AI can do — but how your organization is set up to do it well.
Related Experience
Related Content
The content herein reflects the views and opinions of Summit Partners and is intended for executives and operators considering partnering with Summit Partners. The information herein has not been independently verified. In recent years, technological advances have fueled the rapid growth of artificial intelligence (“AI”), and accordingly, the use of AI is becoming increasingly prevalent in a number of sectors. Due to the rapid pace of AI innovation, the broadening scope of potential applications, and any current and forthcoming AI-related regulations, the depth and breadth of AI’s impact - including potential opportunities – remains unclear at this time. Information herein is as of May 20, 2025.
Get the Latest from Summit Partners
Subscribe to our newsletter to stay up to date on our partners, portfolio, and more.